
- #The royal order of adjectives chart archive
- #The royal order of adjectives chart full
- #The royal order of adjectives chart registration
- #The royal order of adjectives chart trial
#The royal order of adjectives chart full
To assess the accuracy of the algorithm that predicted author affiliation, we selected a random sample of 250 abstracts and manually checked the affiliation of each one of the authors in the full manuscript and compared these results with the algorithm’s classification. Publications in which the algorithm found none of the patterns to classify an author, or found an author with affiliations to both industry and academia or government, were excluded from the analysis. An abstract was classified as industry-authored when any of the authors in the publication had an industry affiliation. The probability was subsequently used in a greedy cluster algorithm to group all papers by an author.Īn abstract was classified as non-industry-authored when all authors of the publication had academic or government affiliations. The classifier was trained on a set where positive cases were identified using author e-mail addresses (only available for very few authors), and negative controls cases were identified based on mismatch in author first name. The probability was estimated using a random forest classifier using these features as input: length of author name, author name frequency in Medline, similarity in MeSH terms, words in the title or words in the abstract, whether the paper was in the same journal, overlap of other authors, and time between publication in years. Because there are no unique identifiers for authors in PubMed, we used an author name disambiguation algorithm similar to Authority, which models the probability that two articles sharing the same author name were written by the same individual. We assumed that if an author had a particular affiliation in one manuscript, that author would also have that affiliation in any other manuscript written by that author in the same year. Appendix 1 contains the complete list of patterns used for the abstract classification.įor the abstracts not included in PubMed Central, we developed an algorithm to predict the affiliation of the authors.
#The royal order of adjectives chart archive
PubMed Central is a free full-text archive of biomedical journals and therefore lists the affiliations of each one of the authors of a manuscript. Because the PubMed affiliation field contains the affiliation of only one of the authors and therefore could not be used as conclusive evidence in papers written by multiple authors, we supplemented the search for the authors’ affiliation, using PubMed Central ®. To determine the affiliation of an author, the affiliation field in PubMed was used to scan for word patterns indicating an industry (e.g., “janssen”, “johnson & johnson”), academic affiliation (e.g., “university”, “school”) or government (e.g., “centers? for disease control”, “u\\.?s\\.? agency”). Studies were classified as industry-authored or non-industry-authored (academia and government), depending on the affiliation of the authors, using an automated algorithm.
#The royal order of adjectives chart trial
We studied the vocabulary used to report trial results and compared it between two authorship groups (industry versus non-industry).

The language used to describe trial results could also affect perceptions of the efficacy or safety of health interventions as well as the quality of the study. In this type of bias, called spin bias, the reader is distracted from the non-significant results. For example, an intervention can be portrayed as beneficial in the publication despite having failed to differentiate statistically from placebo. How trial results are described in publications may influence the reader’s perception of the efficacy and safety of interventions.
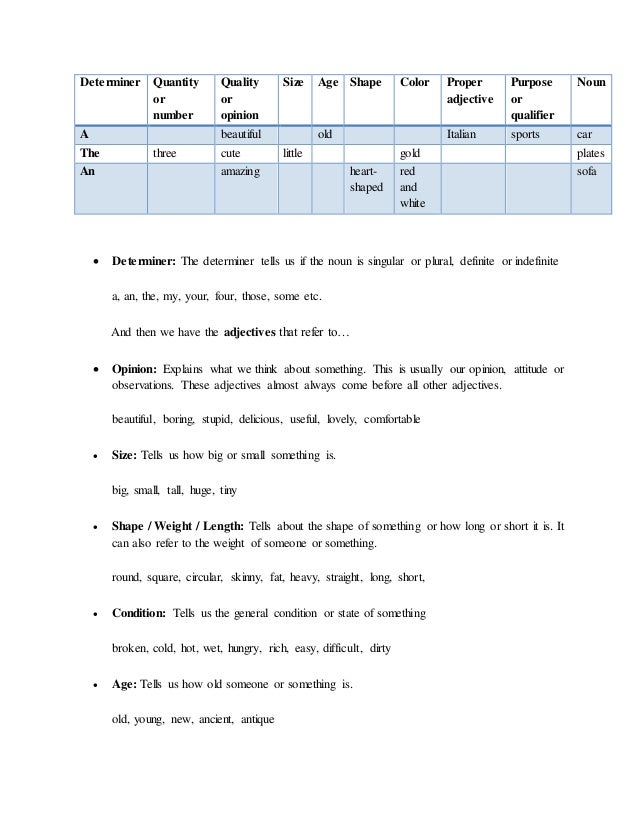

#The royal order of adjectives chart registration
In addition, mandatory registration of clinical trials and mandatory publication of trial results are strategies implemented to diminish the impact of publication bias. The CONSORT initiative has led to improvements in the quality of reporting of trial results.

Inadequate reporting of trial results limits the ability of the reader to assess the validity of trial findings. Publication of only studies that show benefit, known as publication bias, leads to overestimation of the efficacy of interventions. Major impediments to such understanding include selective reporting of trial results and inadequate reporting of trial results. Accurate understanding of the efficacy and safety of health interventions is crucial for public health.
